Kayıp Veri İle Başa Çıkma Yöntemlerinin Yapısal Eşitlik Modellerine Etkisi
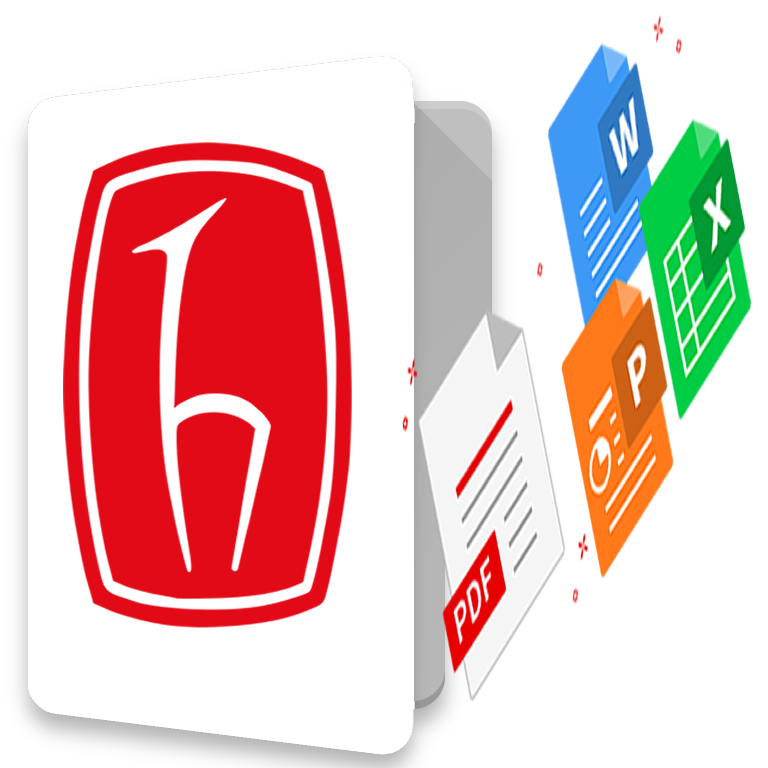
View/ Open
Date
2022Author
Karaman, Haydar
xmlui.dri2xhtml.METS-1.0.item-emb
Acik erisimxmlui.mirage2.itemSummaryView.MetaData
Show full item recordAbstract
The purpose of this research is to investigate three missing data handling methods; listwise deletion, likelihood methods (FIML and EM), and multiple imputation (PMM, CART, NORM) techniques in structural equation models (SEM) under various conditions. These conditions are sample sizes (100, 500, 1000), missing value rates (5%, 10%, 20%), missing value mechanisms (MCAR, MAR), distributions (normal and skewed), and data structure (middle and robust level). This simulation study generated a three-factor SEM model with nine indicators by population correlation matrix. The total number of conditions is 72, and the results are aggregated over 100 replications. Standard error and bias were investigated on eight outcomes of YEM results such as model fit indexes, factor loadings, and path coefficients. Results indicated that listwise deletion with all working conditions was found the most bias and the biggest standard error. The least bias and standard error were found under the MCAR mechanism, normal distribution and robust data structure conditions. FIML and MI methods generally performed the same level, but MI methods failed to perform under 100 sample size, 20% missing data rate, skewed distribution and MAR mechanisms. FIML and MI methods outperformed for goodness of fit indexes however rate of error increased when data skewed. EM methods produced larger error and bias for factor loadings and path coefficients than modern missing handling techniques. Hence, this study recommends using MI to handle missing ordinal data in SEM; unless the missingness is large, the sample size is small, and the distribution is skewed.