Derin Öğrenme Tekniklerini Kullanarak Rgb-D Nesne Tanıma
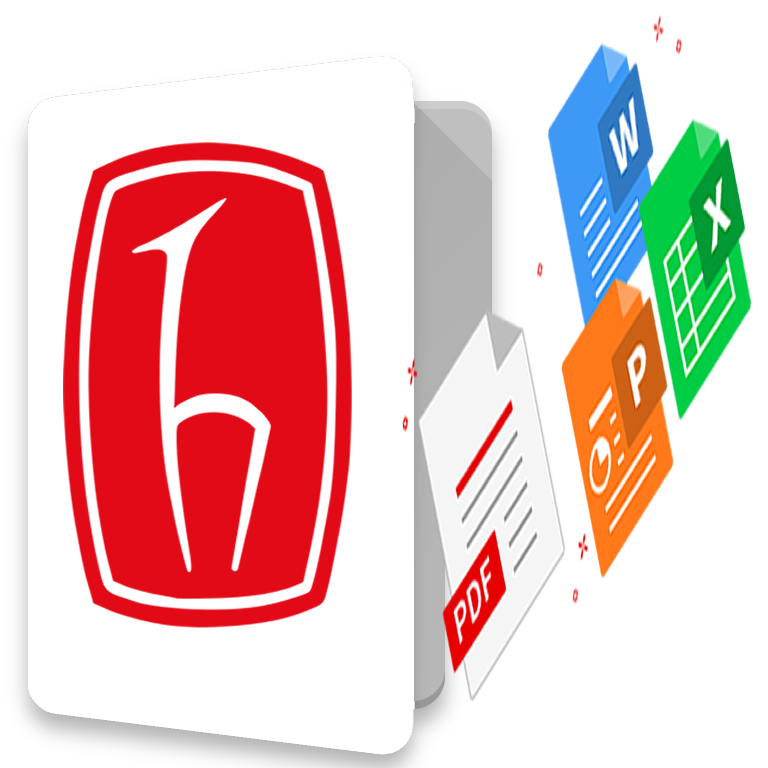
View/ Open
Date
2018Author
Çağlayan, Ali
xmlui.dri2xhtml.METS-1.0.item-emb
Acik erisimxmlui.mirage2.itemSummaryView.MetaData
Show full item recordAbstract
Object recognition is one of the basic and challenging problems of computer vision. With the widespread use of RGB-D sensors such as Microsoft Kinect, which provides rich geometric structured depth data along with RGB images, RGB-D data have emerged as very useful resources for solving fundamental computer vision problems. Particularly in the field of robotic vision, an object recognition task using such data plays an essential role in the interaction of a robot with its surrounding environment and the capability of its visual comprehension. On the other hand, the tremendous progress in deep learning techniques over the last decade, has led to a significant increase in object recognition performance.
In this thesis, several studies on RGB-D object category recognition using deep learning techniques are presented. In these studies, convolutional neural networks (CNN) and recursive neural networks (RNN) are employed. In the first phase of the thesis, an empirical analysis for RGB-D object recognition based on a two-layered shallow architecture with an RNN layer and a CNN layer in which the convolution filters are learned in an unsupervised manner is presented. In accordance with the different characteristics of RGB and depth data, effective model settings and parameters are investigated in this shallow model that learns deep features in a feed-forward manner without backpropagation algorithm. In the next phase of the thesis, various volumetric representations are defined in order to make better use of the rich geometric information stored in the depth data and recognition is carried out with 3-dimensional CNN architectures that take these volumetric representations as inputs. To this end, depth data are represented by 3D voxel grid representations and a suitable 3D CNN model is presented for these representations by experimentally investigating among many different alternatives. In the last part of the thesis, a new approach based on transfer learning for RGB-D object recognition is presented. To this end, firstly, a pretrained CNN model is used to extract features from different layers for RGB and depth data. Then, these features are transformed with RNN structures to map to higher-level representations. Finally, the representations derived from different levels are fused to produce a final vector expressing the holistic object image.
The proposed works are analyzed with extensive experiments performed on the well-known datasets for RGB-D object recognition. The proposed works produce successful results that confirm the main objectives and the results are higly competitive with the related studies.