ON THE YIELD CURVE FORECASTING: APPLICATIONS OF CONVENTIONAL AND NON-CONVENTIONAL TECHNIQUES
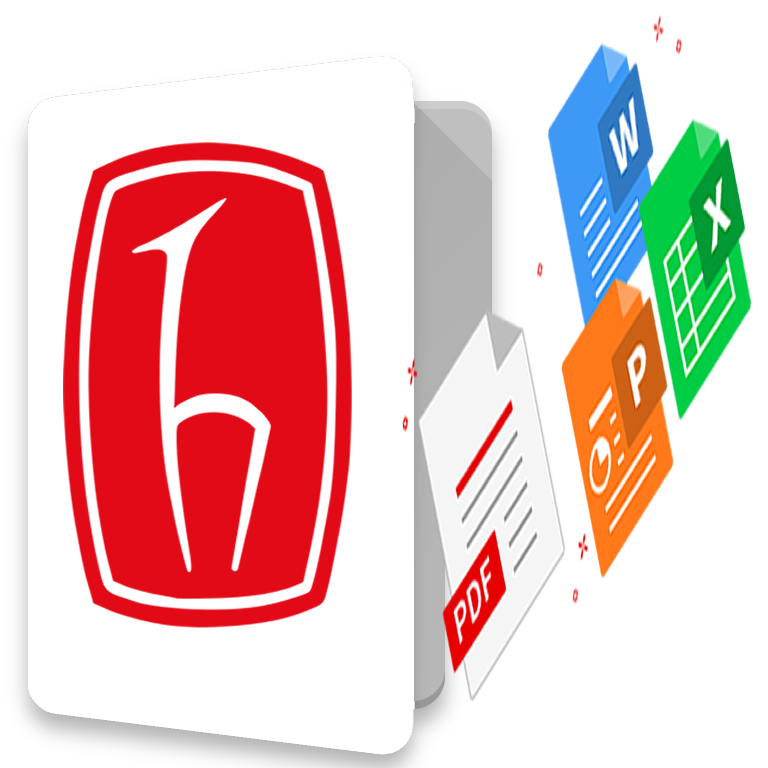
View/ Open
Date
2025Author
GENÇSOY, Hakan
xmlui.dri2xhtml.METS-1.0.item-emb
Acik erisimxmlui.mirage2.itemSummaryView.MetaData
Show full item recordAbstract
Forecasting the yield curves of government debt securities observed in the market is very crucial for both economic and financial units. Diebold and Li (2006) show that the AR(1) model makes the best forecasts with the Dynamic Nelson Siegel approach. Many researchers have later used this approach with different data and models. The differences and new approaches that emerge in the literature reveal the need for a more comprehensive study for yield curve forecasts. In this thesis, we forecast the yield curves of government bonds of G-7 countries, excluding Japan, for the period 2010-2022 using the Dynamic Nelson Siegel approach. We used conventional, non-conventional models, ensemble learning, and forecast combination models. Artificial Neural Networks (ANN), which have been frequently used in recent years and can investigate the data structure more effectively, have been used in the thesis. In the thesis, unlike the literature, we smooth the decay parameter (λ) of the Nelson-Siegel Model with the high-frequency Hodrick-Prescott Filter except for the United States. Thus, the λ parameter, like other factors, is included in the variables to be forecasted. In this thesis, we increase the flexibility of the yield curves, and the results of the forecasts are more meaningful. The results of the thesis are as follows. The yield curve fits the yields better with the float λ compared to the constant. In this thesis, we investigate models that make better forecasts than the random walk model, we show that although individual models mostly fail, successful forecasts can be easily made with forecast combination models we obtain from individual models. We also conclude that different ARIMA models can be used instead of AR(1), and that ensemble learning and forecast combination approaches can improve the forecasts of individual models. We conclude that ANN models are unstable in making successful forecasts.