Development of A Deep Learning Approach for 3Dimensional Point Cloud Classification
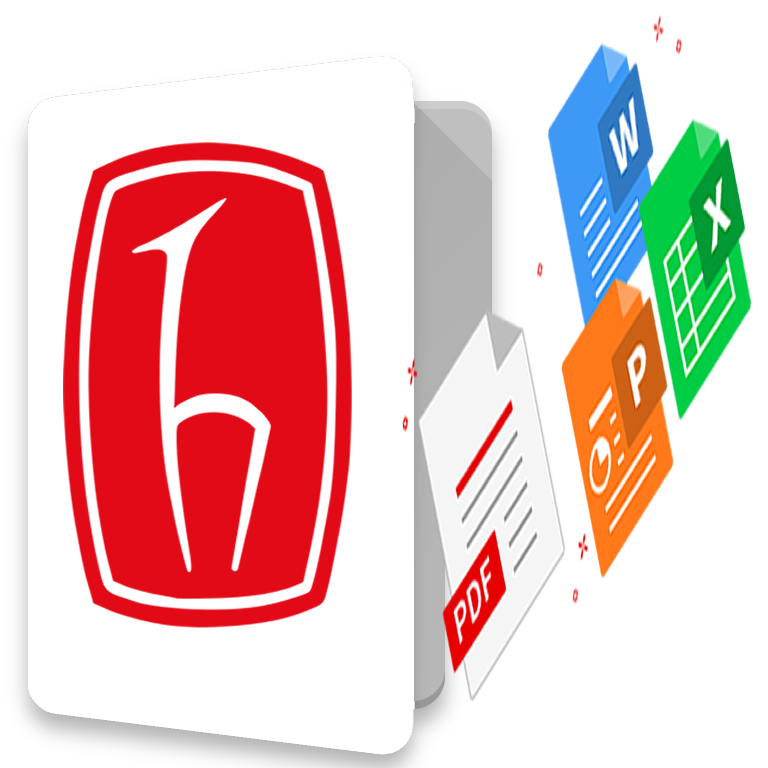
View/ Open
Date
2024-09Author
Sevgen, Eray
xmlui.dri2xhtml.METS-1.0.item-emb
6 ayxmlui.mirage2.itemSummaryView.MetaData
Show full item recordAbstract
3D point cloud classification is a crucial step in point cloud processing, as it serves as
the foundation for subsequent processes that rely on the classification results. With the
increasing volume of 3D point cloud data in recent years, the need for automation in 3D
point cloud classification has grown significantly. Automation is essential for reducing
errors, eliminating additional costs, and providing more robust processing pipelines. As
a result, automating 3D point cloud classification has become both a necessity and an
active area of research. Over the past decade, machine learning approaches have gained
popularity in nearly every field of science and engineering. Similarly, 3D point cloud
processing has also adopted machine learning methods. In recent years, studies on 3D
point cloud classification have largely focused on machine learning models. Among these,
neural networks, a subfield of machine learning, have emerged as a prominent approach,
especially deep learning models. In this thesis, 3D point cloud classification is first performed
using a recent state-of-the-art machine learning classifier. Then, manually extracted feature
sets are incorporated into deep learning models, and an additional neural network layer is
designed to process these features instead of directly feeding them to the model. Finally, a
global feature aggregation module is proposed to capture more contextual information and further improve the results. These three methods were trained and tested on several 3D point
cloud datasets: ISPRS3D, Paris-Rue-Cassette, Paris-Rue-Madame, H3D, and Toronto3D.
The datasets are publicly available and used in previous studies as a benchmark dataset.
The datasets have various different properties; three of them were collected using mobile
mapping systems, one using an airborne laser scanner, and another using an unmanned
aerial vehicle-borne laser system. The datasets vary in point densities and the number
of classes. According to the results obtained from each proposed models, all methods
achieved over 80.0% overall accuracy. Notably, the machine learning model produced
promising results, even competing with deep learning-based methods. Although deep
learning methods improved classification metrics, satisfactory results were achieved only in
large-scale datasets. With further refinements, both deep learning models have the potential
to make significant advancements, as indicated by recent literature.