Koşullu Otoregresif Mekânsal - Zamansal Modeller ile Yabancı Dil Sınavı Puanlarının İstatistiksel Analizi
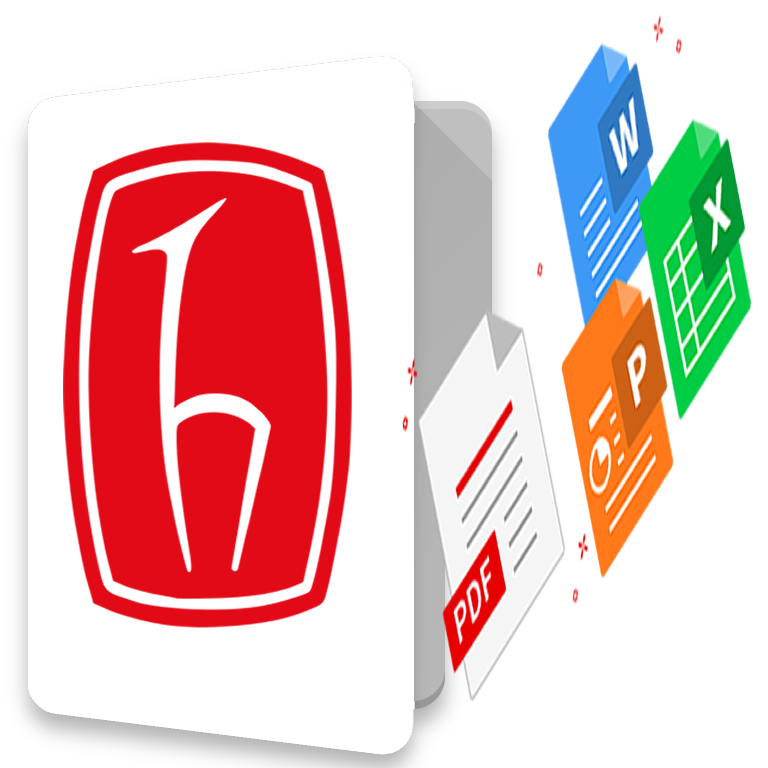
View/ Open
Date
2024-05Author
Dinarcan, Sercan
xmlui.dri2xhtml.METS-1.0.item-emb
Acik erisimxmlui.mirage2.itemSummaryView.MetaData
Show full item recordAbstract
Spatial-temporal data consist of observations occurring at a specific time or time period, with specific coordinate values and a specific geographic reference system for these coordinate values. The most important feature of this data is that observations collected at close times from geographically close units tend to be more similar than those collected at more distant units and times. Spatial and temporal similarities in the data naturally lead to spatial-temporal relationships in the variables. Therefore, when modeling spatial-temporal data, the spatial locations of the units that generate the observations, the temporal variations in the observations of these units, and their interrelationships to each other should be used. When spatial-temporal data are modelled with models such as Generalized Linear Models (GLM), the residuals contain spatial and temporal autocorrelation. This leads to the violation of the model assumptions. Spatial-temporal models remove the spatial and temporal autocorrelation in the residuals and then stable models are built. In this thesis, it is aimed to determine the spatial-temporal effects, similarities and variations in the scores of the Foreign Language Test (FLT) conducted between 2015 and 2020 and to reveal the factors affecting the success. To achieve this aim, the averages of the FLT scores were modelled with spatial-temporal models on the basis of provinces of Turkey, which are the largest spatial unit formed by the administrative divisions of Turkey. Moran's I test showed that the averages of the FLT provincial scores have spatial autocorrelation. Spatial-temporal similarities and differences in the data were presented visually using various mapping techniques. Factors that are considered to affect FLT scores were included in the model and the impacts of these factors on FLT provincial success were evaluated. In this study, conditional autoregressive spatial-temporal models are employed. These models are generalised linear mixed models, which are developed by adding spatial-temporal random effects to generalised linear models. Spatial-temporal random effects can be defined in different forms and have conditional autoregressive prior distributions in accordance with their forms. Generalised linear mixed models based on the forms of random effects are modelled hierarchically using a Bayesian approach. This thesis is the first study in which FLT data were analyzed statistically with spatial-temporal models. In order to apply the conditional autoregressive spatial-temporal models analyzed in this thesis to the FLT data, the CARBayesST package (version: 3.2.1) in the open-source R programme was used. The best fitting model was selected by using the model fit criteria such as the deviation information criterion. The averages of FLT scores of the provinces for all years were estimated using the best fitting model, and the estimated averages of FLT scores were presented using mapping techniques. The most and least successful provinces were determined. Modeling results and spatial-temporal effects on FLT were discussed in detail.