Gudermannian Kayıp Fonksiyonu ve GudermannianBoost İkili Sınıflandırma Yöntemi
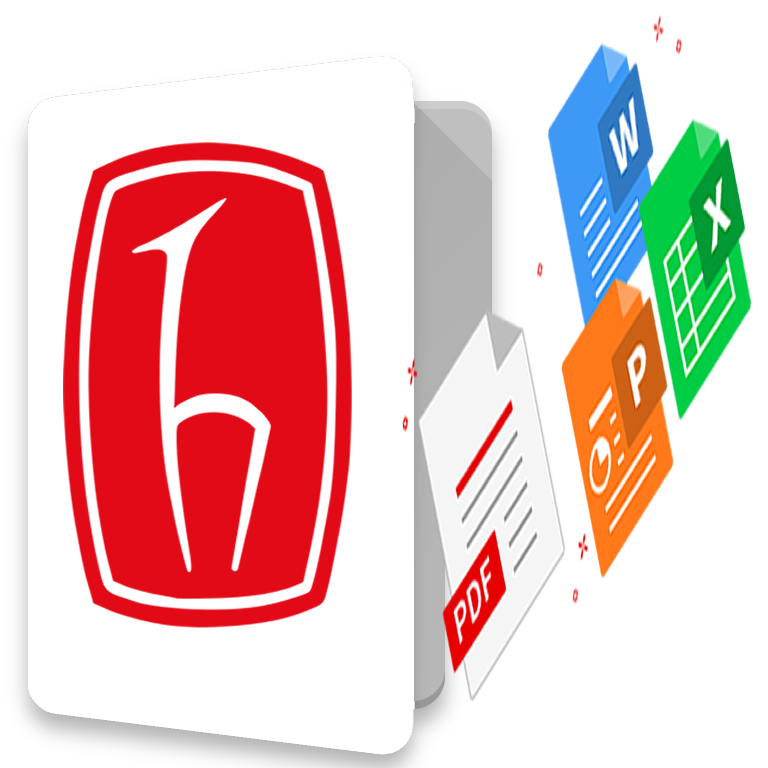
View/ Open
Date
2016-12Author
Toka, Onur
xmlui.mirage2.itemSummaryView.MetaData
Show full item recordAbstract
In this study, a robust loss function and binary boosting classification method are proposed.
The purpose of classification methods is to obtain classifier function with generalization ability, i.e., high prediction performance. Boosting methods as iteratively algorithms, which include a loss function and weak classifier, are a way of predicting class labels of given inputs. Loss function is the way of penalizing conditional risk in boosting algorithm. While most of loss functions only penalize the misclassification, some robust loss functions give penalties not only large negative (misclassification) margin but also large positive (accurate classification) margin in order to get more stable classifiers. Robust loss functions stand up to outliers and contaminated part in training data. Therefore, classifiers are the methods which display high prediction performance in testing part.
This study reports brief information about loss functions, robust loss functions and their properties. In addition, there is a correction to ensure statistical consistency on the algorithm of TangentBoost, one of the methods having all properties of robust loss functions. Finally, in order to get more stable classifiers, Gudermannian loss function, which gives more penalties for both small positive and small negative margin than Tangent loss function does, and GudermannianBoost as a corresponding binary classification boosting method are proposed. The advantages of GudermannianBoost method are discussed based on the applications of some spesific simulation scenarios and some real datasets.