Learning Heuristics Towards Solving Combinatorial Optimization Problems
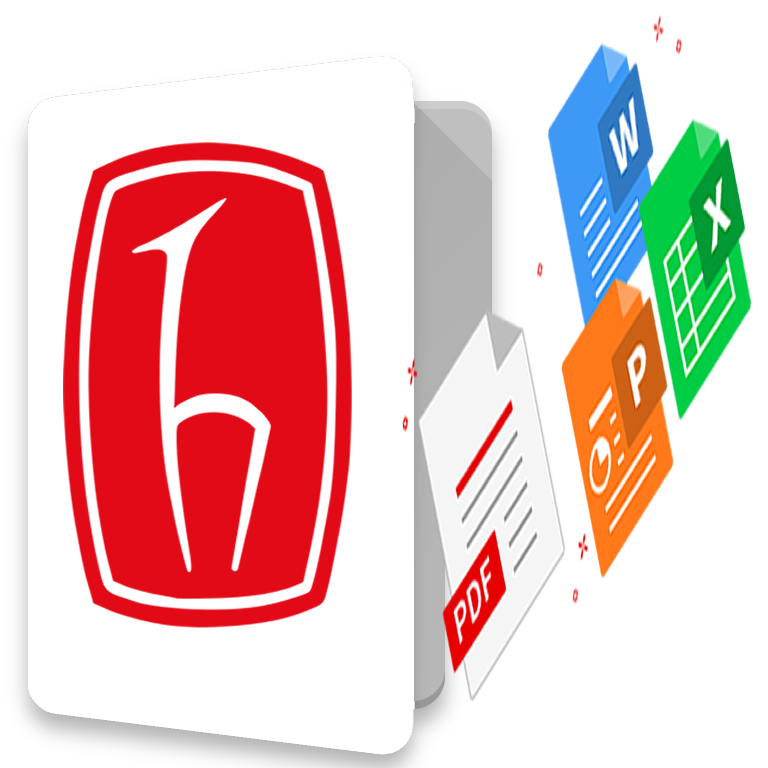
View/ Open
Date
2022Author
Taşdemir, Ali Baran
xmlui.dri2xhtml.METS-1.0.item-emb
Acik erisimxmlui.mirage2.itemSummaryView.MetaData
Show full item recordAbstract
We introduce a learning framework towards the approximation of the maximum clique enumeration problem. We make use of node classification to eliminate the least likely nodes to be in a maximum clique and reduce the input size. We study the effect of using various node representations on this learning process. Our main contribution is an extensive study on comparing the performance of different node representation methods, ranging from graph embedding algorithms, such as Node2vec and DeepWalk, to representing nodes with higher-order graph features comprising local subgraph counts. We also present an effective method based on feature elimination to reduce the computation time even further. Finally, we provide tests on random graphs to show the robustness and scalability of our results.