Solunum Seslerinin Evrişimsel Sinir Ağlarıyla Sınıflandırılması
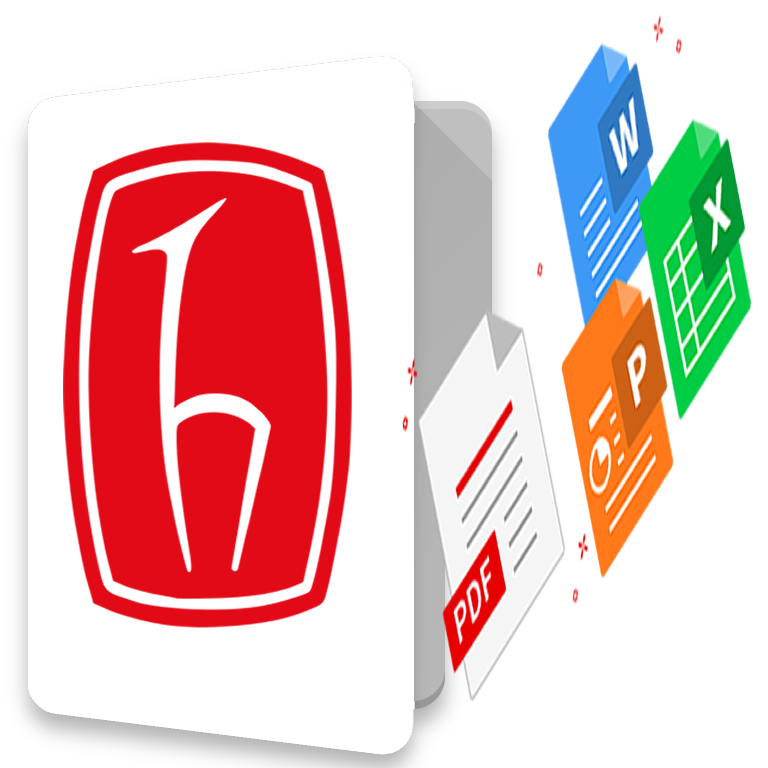
Göster/ Aç
Tarih
2022-06Yazar
Cinyol, Funda
Ambargo Süresi
Acik erisimÜst veri
Tüm öğe kaydını gösterÖzet
Physicians use stethoscope in clinical examination because it is both non-invasive and easily accessible and inexpensive diagnostic and detection tool. However, at this stage, many negative reasons such as ambient noise, hearing ability of the physician, age, etc. make the diagnosis difficult. In addition, respiratory sounds contain important information at frequencies close to the lower limits of human hearing ability. It is important to record these sounds with an electronic stethoscope in order to present a more quantitative approach. In this thesis, 294 lung sounds collected in a clinical setting were classified for 3 different sound groups (Normal, Crackle, Rhonchi).
Although many studies have been carried out on the classification of respiratory sounds with artificial intelligence methods, the inadequacy of the data used has prevented the creation of a solid architecture. In order to cope with this shortcoming, the ICBHI 2017 dataset was created and the classification studies of lung sounds gained momentum.
Convolutional Neural Networks (CNNs); It is a method frequently used in breathing sound classification of Deep Neural Networks, which is used in many areas such as image and video recognition, image classification, natural language processing and medical image analysis. In CNNs, softmax function is generally used for classification in the last layer. However, instead of using only the softmax function, the use of Support Vector Machines (SVM) is also included in the literature. In this thesis, both the architectures created by using Softmax in the last layer of CNN (CNN-Softmax) and the architectures in which CNN is used together with SVM in the last layer (CNN-SVM) were created. In addition, VGG16-CNN-Softmax and VGG16-CNN-SVM architectures were created by combining the CNN-Softmax, CNN-SVM models created with the VGG16 model with the help of transfer learning. These architectures have also been compared with proven learning transfer methods in the literature (VGG16, DenseNet201, InceptionV3 and ResNet101). These architectures have also been compared with proven learning transfer methods in the literature (VGG16, DenseNet201, InceptionV3 and ResNet101). The highest classification accuracy obtained as a result of 10-fold cross validation by separating the training and test data by 80%- 20% was achieved with the VGG16-CNN-SVM model.
The classification success metrics of the proposed method are obtained as respectively; ROC AUC Score: 88.4%, Maximum Accuracy: 83%, precision = 82%, recall= 83% and F1 score = 82%.
In addition, for three groups of voice data, Normal 84%, Crackle 80% and Rhonchi were classified with 86% accuracy, respectively.