CUDA Based Real Time Implementation Of Region Covariance Descriptors
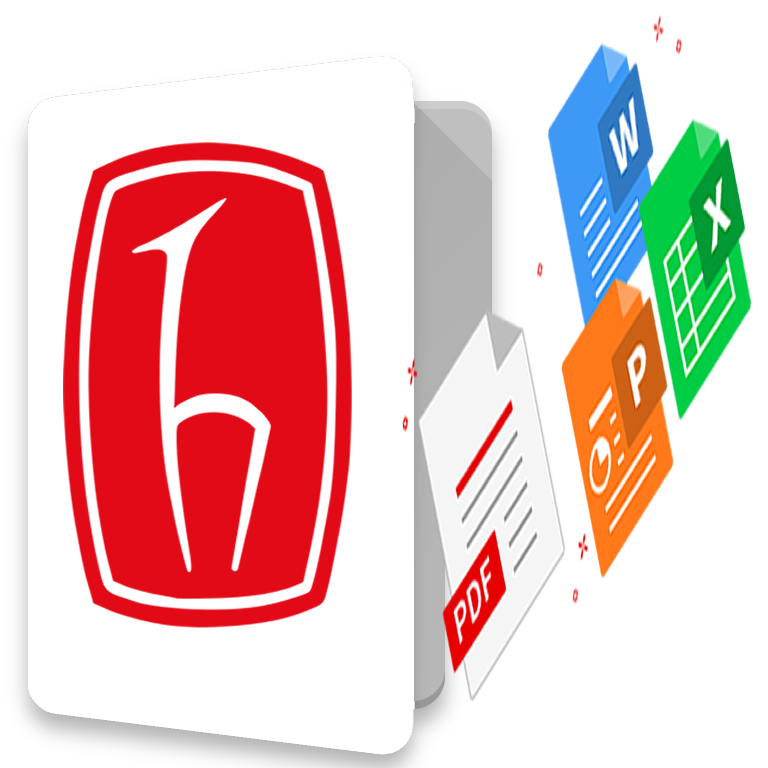
Göster/ Aç
Tarih
2019-09Yazar
Asan, Muhammet Ali
Ambargo Süresi
Acik erisimÜst veri
Tüm öğe kaydını gösterÖzet
Computation time of computer vision applications is critical for real-time applications such as video processing. While applied methods achieve real-time performance, it is also vital to allow processors to work on other tasks. In order to use in real time video processing tasks, an algorithm should achieve 30 frames per second. In computer vision, the input data mostly consists of two dimensions thus running time of most applications are proportional to the square of input size.
To allow a computer system to process images, the computer must be able to understand the image. Image descriptors play an important role to help computers with understanding of images. Extracting features from image, classification, recognition, comparison of images becomes possible by using these features with image descriptors. In this study, a parallel implementation of a robust image descriptor called Region Covariance Descriptor on GPU using CUDA is given. While the serial algorithm is not enough for real-time processing, applied parallel implementation achieves real-time performance.
In this study, parallel and asynchronous computation of region covariance descriptors on GPU both allows CPU to perform other tasks and achieves 30 images to be processed in a second. Region Covariance Descriptor which is introduced in object recognition and image classification application at first makes it possible to use covariance of basic image features like color, gradients as a robust descriptor. This descriptor is also applied to several image filtering and object tracking problems as well. As region covariance descriptors do not live in Euclidean space, traditional vector distance measurements methods can not be used to compare them. Instead, a symmetric positive definite matrix comparison method which is based on generalized eigenvalue of two matrices is used to compare covariance matrix. But because this method is complex, porting the algorithm to GPU is difficult and execution time takes longer. After our reviews on existing methods, we have replaced traditional covariance matrix comparison method with a metric which is robust, easy and fast to compute on GPU. In this study analysis of these two distance metric calculation methods is also discussed.
One of the main contributions of this work is that in this study an existing CUDA based integral image computation on GPU is replaced by a novel approach which works faster and uses less memory than the existing method. We should note that the proposed approach does not leverage any development in hardware between two works.
Bağlantı
http://hdl.handle.net/11655/9409Koleksiyonlar
Künye
Muhammet Ali Asan, CUDA Based Real Time Implementation Of Region Covariance Descriptors, Yüksek Lisans Tezi, Hacettepe Üniversitesi, 2019)Aşağıdaki lisans dosyası bu öğe ile ilişkilidir: