Sentinel-1 ve Sentinel-2 Verilerinden Tarımsal Ürün Sınıflandırması İçin Makine Öğrenme Algoritmalarının Karşılaştırılması
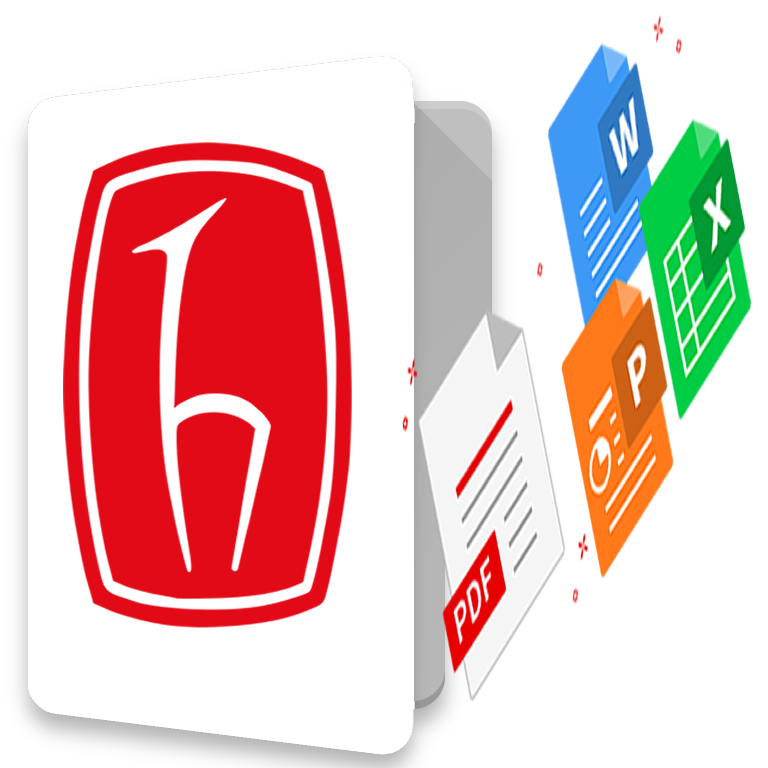
Göster/ Aç
Tarih
2019-02Yazar
Dizdaroğlu , Timuçin
Ambargo Süresi
Acik erisimÜst veri
Tüm öğe kaydını gösterÖzet
In this thesis, machine learning algorithms were compared for object-based classification of multi-date Sentinel-1 SAR and Sentinel-2 optical satellite images of agricultural summer crops grown in Gediz plain. The machine learning algorithms used in the study were DT (Decision Tree), RF (Random Forest) and SVM (Support Vector Machines). In addition to the original bands of Sentinel-1 and Sentinel-2 satellite images, NDVI (Normalized Difference Vegetation Index) bands and texture (dissimilarity, entropy, homogeneity, angular second moment, correlation and correlation) bands were also calculated and used in the classification process.
Eight images selected between April and November 2017 of the Sentinel-1 and Sentinel-2 satellite images have been used in the study. The selected dates of Sentinel-1 satellite images were 13 April, 1 May, 1 June, 30 June, 31 July, 5 September, 11 October and 16 November 2017, while the dates selected for Sentinel-2 satellite images were 10 April, 3 May, 2 June, 2 July, 1 August, 7 September, 10 October and 16 November 2017.
In the first step of the study segments were created by performing multi-resolution image segmentation. Features were calculated for each of the generated segments. The classification process was carried out through object-based DT, RF and SVM algorithms. Different combinations of Sentinel-1, Sentinel-2 original bands, texture bands and NDVI bands were used in the classification process. When only the original bands are used in classification, the highest classification accuracy for Sentinel-1 images was obtained by the RF algorithm (90.48%). Similarly, for the Sentinel-2 images, the highest classification accuracy was also calculated by the RF algorithm (90.96%). When the images of both satellites were used together (Sentinel-1 + Sentinel-2 + NDVI), the highest accuracy (%93,37) was given by the SVM algorithm and this was the highest accuracy value obtained in this study.
Based on the results, when the combination of features were evaluated, the texture and standart deviation bands did not increase the classification accuracy, whereas they decreased the accuracy. The reduction in the accuracy varies between 1% and 5% depending on the combination of features and the classfication algorithm used. On the other hand, when Sentinel-1 original bands were used in combination with Sentinel-2 bands, the positive effect of Sentinel-1 bands on the classification accuracy was approximately 1-2% in all combinations. In addition, in the feature combinations where Sentinel-1 bands were used alone, very high accuracy values have been calculated. Finally, when NDVI mean values were considered, it was seen that NDVI bands have increased the accuracy in all feature combinations and algorithms. Furthermore, high accuracy values were achieved when the classification was carried out using the NDVI bands only.The overall accuracy of 92,65% was calculated when classification was carried out using the RF algorithm and NDVI bands only.