Türkçe Ağızların Tanınmasında Derin Öğrenme Tekniğinin Kullanılması
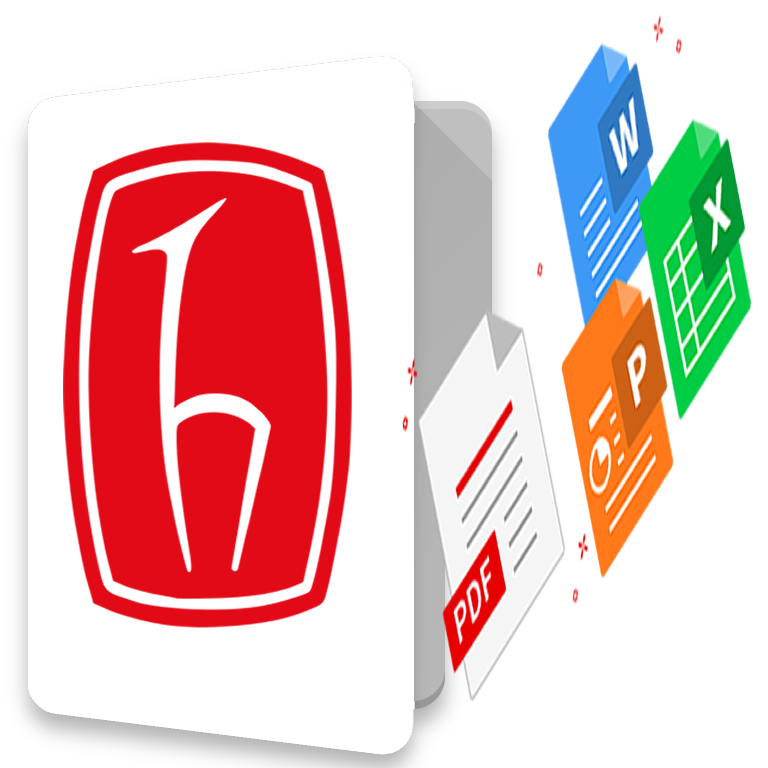
Göster/ Aç
Tarih
2019-02Yazar
Işık, Gültekin
Ambargo Süresi
Acik erisimÜst veri
Tüm öğe kaydını gösterÖzet
Automatic speech recognition systems are used to translate speech sounds into text. The performance of the automatic speech recognition system in any language is dependent on the speaker gender and emotion as well as dialects that are variants of the language. Dialects are the speech forms that are similar to each other in the same geographic region as the utterance and lexical structure. With these characteristics, dialects are separated from each other. The aim of the dialect recognition is to identify the humans’ dialect from their speech. Following the recognition of the dialect, it is known that the performance of the speech recognition system is enhanced by adapting the language and acoustic models to this dialect. Furthermore, identifying spoken dialect from speech can be used as a preprocessing step in voice response systems, or it can help to obtain a clue in forensics.
The modeling techniques used in dialect recognition are intended to model information in different language layers. Features in the acoustics, phonotactic and prosodic layers give important information that specific to the dialect. Phonetic differences of speech can be determined by examining their spectral features at the physical level. Features such as classical Mel Frequency Cepstral Coefficients (MFCC) and Log mel-spectrogram are used for this purpose. Phonotactic corresponds to the rules of coexistence of phonemes in a language/dialect. Phoneme sequences and the frequency of this sequence vary from dialect to dialect. Phoneme sequences are obtained by phoneme recognizers and then phoneme distributions are extracted using language models. Prosody is the auditory features of speech such as intonation, stress and rhythm. It is known that these features play a key role in the human perception of speech. These perceptual features are extracted by measuring the fundamental frequency (pitch), energy and duration at the physical level and converted into appropriate parametric representations.
In recent years, Convolutional Neural Networks (CNNs) have been frequently used particularly in image and speech recognition since deep neural networks become popular. In addition, Long Short-Term Memory (LSTM) recurrent neural networks are widely used in sequence classification and language modeling problems. LSTM neural networks are more successful in modeling long-term context information than n-gram models.
Dialects spoken by people living in different regions of Turkey are separated from each other in terms of features mentioned above. From this perspective, in this thesis, acoustics, phonotactic and prosodic features were used to classify Turkish dialects with CNN and LSTM neural networks. For this purpose, a Turkish data set consisting of Ankara, Alanya, Kıbrıs and Trabzon dialects was formed. The proposed methods have been tested and interpreted on the Turkish data set. As a result of the study, it was observed that the methods used gave very good results for Turkish dialect recognition.