MIWGAN-GP: Missing Data Imputation using Wasserstein Generative Adversarial Nets with Gradient Penalty
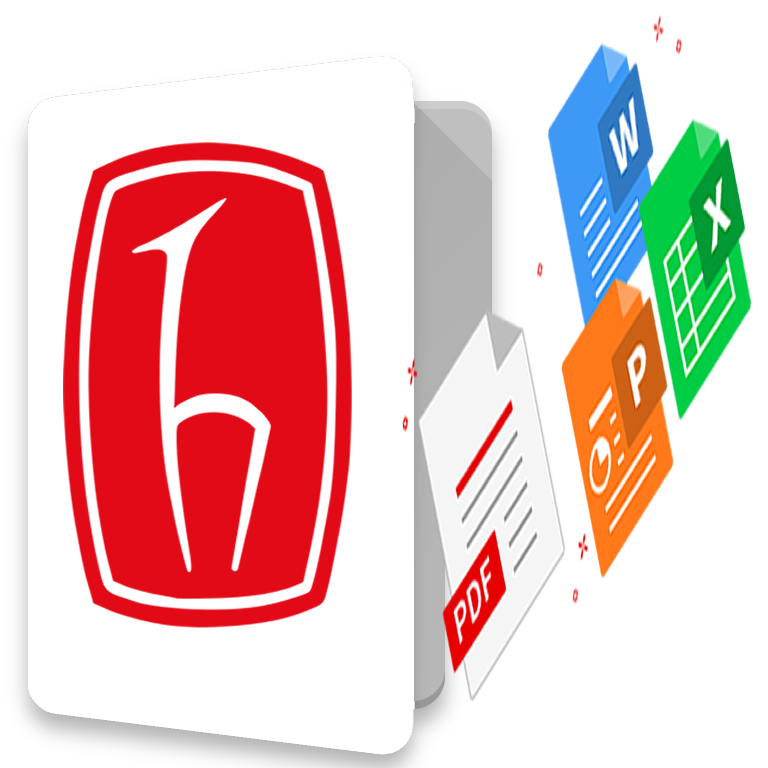
Göster/ Aç
Tarih
2022Yazar
Uçgun Ergün, Ebru
Ambargo Süresi
Acik erisimÜst veri
Tüm öğe kaydını gösterÖzet
The success and dependability of IoT applications are heavily dependent on data quality. Due to hardware problems, synchronization challenges, inconsistent network connectivity, and manual system shutdown, produced data might be missing, erroneous, and noisy. These missing or erroneous values can also occur on health, military and surveillance data and result in errors can also cause important errors in mission systems. If the mission critical system is used in medical domain such missing data problems may affect human life. Hence, Missing values should be imputed appropriately to avoid erroneous judgments in IoT healthcare systems and other critical systems.
In addition, Naive Bayes, K-Nearest Neighbors, Decision Tree and XGboost algorithms are applied in the IoT health sector in this study to show in detail the effect of missing data on the outputs of machine learning algorithms. Following that, we compare different strategies for imputing missing data. The classification methods used were compared both for each defect percentage and with different imputation methods.
In this thesis, a new GAN-based approach is proposed to complete the missing data. The success of the proposed method is compared with classical imputation methods. Error measurements are realized with four different error metrics. In addition, the success of the proposed GAN-based model is demonstrated by applying different classification methods on the data set filled with this method.