Gelişmiş Sinyal İşleme için Verimli Heterojen Paralel Hesaplama
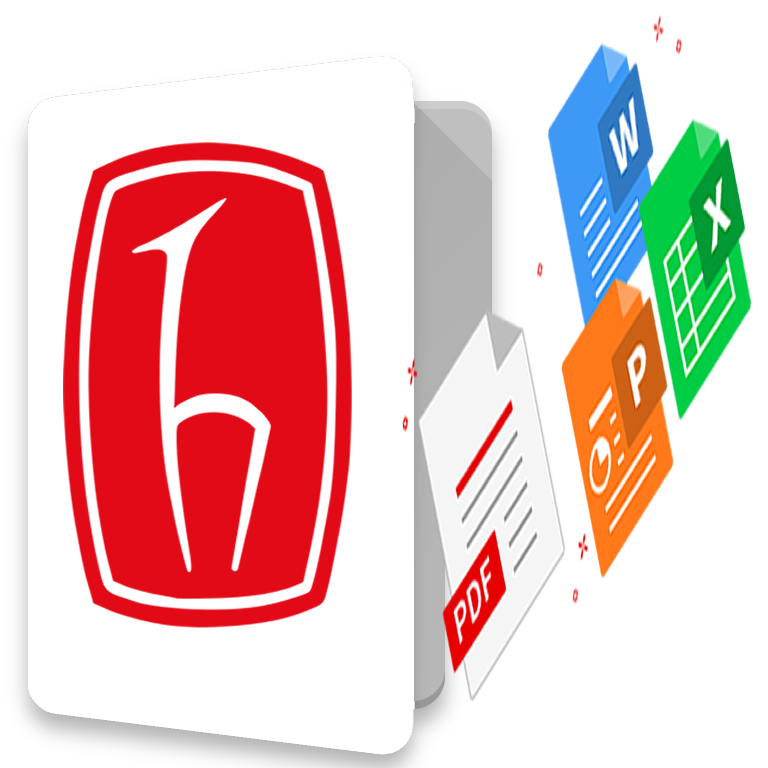
Göster/ Aç
Tarih
2022Yazar
Fişne, Alparslan
Ambargo Süresi
Acik erisimÜst veri
Tüm öğe kaydını gösterÖzet
In this thesis, power-efficient, real-time, parallel applications are designed which aims to reduce increased computational overhead of advanced signal processing algorithms compared to conventional signal processing methods. The bottleneck processing points, memory requirements and computational complexity are analyzed for computations of digital down conversion, machine learning-based target detection and compressed sensing-based direction of arrival estimation in the sensor signal processing architecture. The parallel computation steps are designed as a result of comprehensive analysis. Performance improvements have been achieved for advanced signal processing applications with heterogeneous parallel computing. In machine learning-based target detection, the computational complexity has been reduced by approximately 8.5x with the layer optimizations, and GPU computing provided 7x acceleration compared to CPU parallel version for this application. In the digital down conversion application, real-time down conversion was implemented with 6.5 Watts, leading to extremely low power consumption. For the compressed sensing-based direction of arrival estimation, CPU and GPU-based improvements have been performed to succeed real-time computation requirements. With parallel computations, real-time applications were integrated with 15 Watts power consumption. Thus, an efficient solution has been presented for mobile sensor architectures with low power consumption requirements. According to the hypotheses and the results of the performance tests, this thesis has presented a design concept that contributes to meet the real-time sensor signal processing requirements of edge computing units that need long battery life.