X-ray Görüntülerinde Derin Öğrenme Yöntemleri ile Tehdit Tespiti
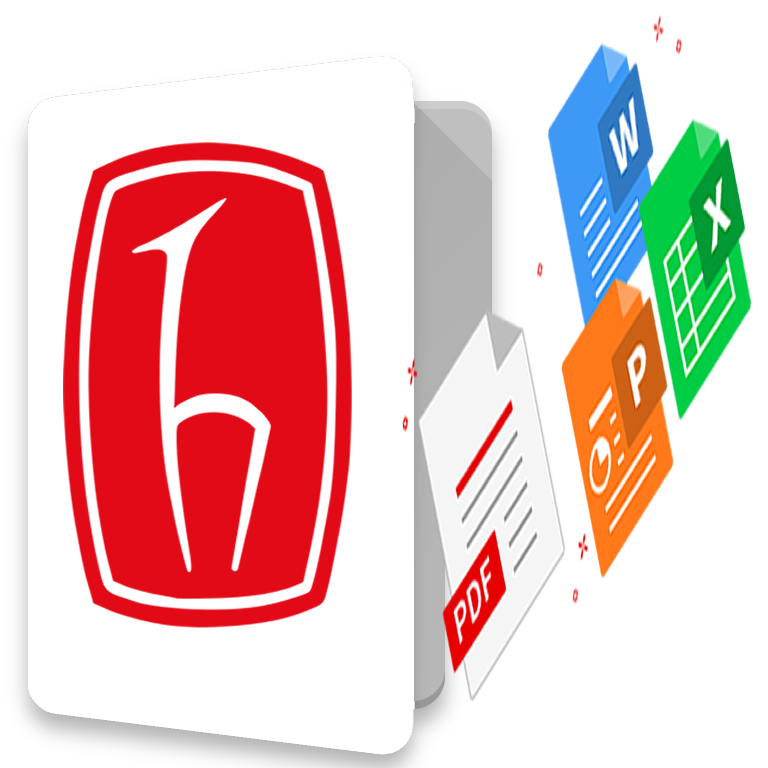
Göster/ Aç
Tarih
2022-01Yazar
Altındağ, Elif
Ambargo Süresi
Acik erisimÜst veri
Tüm öğe kaydını gösterÖzet
In recent years, X-ray imaging equipment are frequently used in crowded and risky environments such as airports, schools and shopping malls to ensure public safety. However, human labor is still needed in places where X-ray imaging techniques are used. This is because the accuracy required to automatically detect potentially threatening objects in X-ray images is not sufficient. For this purpose, studies on the detection of prohibited items that are not allowed to be brought into public area using deep learning techniques have gained accelaration in recent years. In this thesis, threat detection from X-ray images was accomplished using deep learning-based object detection methods and it was aimed to contribute to this field.
In this study, two different datasets, one of which is open source and the other collected within the scope of the study, were used. The X-ray images collected were acquired by a dual-channel X-ray scanner. Dual-channel X-ray scanners use both low and high energy levels. By using two energy levels, additional information such as the atomic number and density of the scanned material is obtained, and with this method, more detailed information about the characteristics of the objects is obtained. Thus, as a result of a scan, we have three images in total which are low-energy, high-energy, and colored X-ray image. It is important to note that a colored X-ray image is obtained by using high-energy and low-energy X-ray images. Since the open-source dataset is more comprehensive than the collected dataset, the object detection algorithms used are primarily trained on this set. In this context, YOLOv3, SSD, and Faster RCNN, which are popular object detection algorithms, were used and the results obtained on the open-source and comprehensive dataset were compared. As a result of the comparison, the training of the collected dataset was done by using the results obtained from the method with the highest performance. This is because the collected dataset contains a small number of images. While transferring to the collected dataset, the few-shot learning method was used and it was investigated how successfully the threats in the collected dataset would be detected with as few images as possible. Another study after the few-shot learning is transferring the best result obtained as a result of training the open-source dataset to the collected dataset with fine tuning. In the last experiment, within the scope of the study, colored, low and high energy images of the collected data set were trained end-to-end in different combinations. The aim here is to observe how successful the information obtained from low and high-energy images will be in detecting threats in a dataset containing a small number of images. When the results were examined, it was seen that the training made with Faster RCNN achieved the highest success on the open source dataset. In the case of transferring these results to the collected dataset, the best success was obtained with the fine-tuning method. In the case where the low and high-energy images of the collected dataset are applied with different combinations, the highest success is achieved when low-energy, high-energy and colored X-ray images are included in the training.