Artıfıcıally Intellıgent Players For Pvp In Mmorpgs
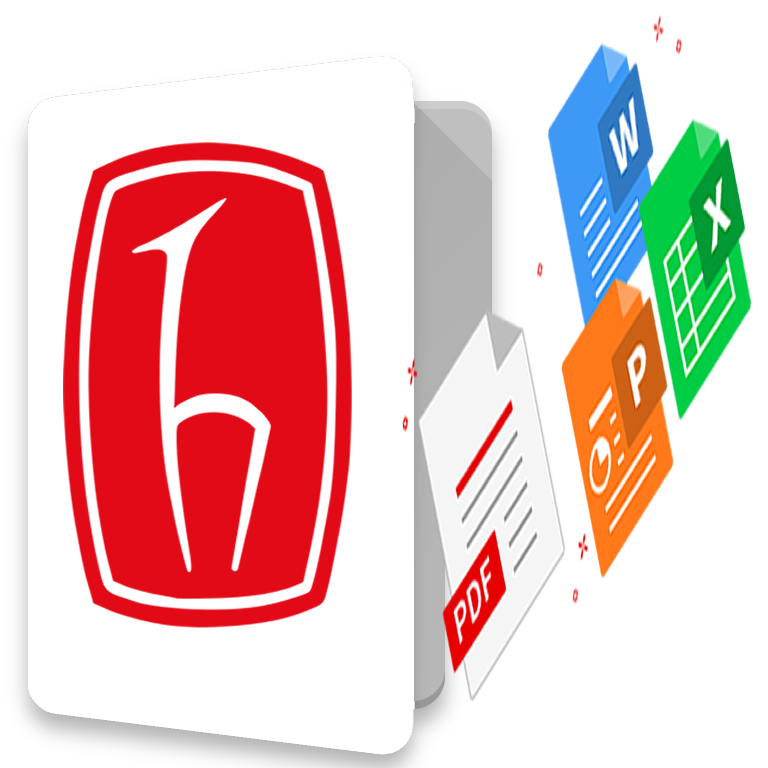
Göster/ Aç
Tarih
2020-12-16Yazar
Karşı, Tarık
Ambargo Süresi
Acik erisimÜst veri
Tüm öğe kaydını gösterÖzet
Massively Multiplayer Online Role Playing Games (MMORPG) are very similar to real life problems since they are complex and partially observable. These games, which have many features such as trade, character improvement, item crafting, questing and player versus player (PvP) battle, have hosted many studies. It is also very suitable for developing and testing artificial intelligence algorithms in terms of the difficulty of the domain, its similarity to real life and abundance of data. In PvPs, the players need to take hundreds of real time actions and develop complex strategies in a partially observable environment. Therefore building an AI for PvPs in MMORPGs constitutes a difficult problem.
With reinforcement learning, a general AI can be developed that can make PvP in MMORPGs. But, designing a reward function manually for reinforcement learning problems in partially observable environments is quite a daunting task. Most of the existing work in the literature aim to accomplish reward shaping by learning from demonstrations or with some kind of human assistance like preferences or rankings. However, for most of the real world problems, it’s nearly impossible to attain such facilities, and even if they are available, they restrict the success of the agent to some human level. In this work, we propose a reward shaping method for PvP in MMORPGs without any demonstrations or human assistance, and compare it with
several manually designed reward functions. We compared manual reward shaping method, which use predefined rewards, with the MCTS reward shaping method, which only exploits the prediction characteristic of a simply crafted Monte Carlo Tree Search planner. PvP results for a single character type showed that MCTS method can be used for reward shaping.
Bağlantı
http://hdl.handle.net/11655/23268Koleksiyonlar
Aşağıdaki lisans dosyası bu öğe ile ilişkilidir: