Learnıng Vısually-Grounded Representatıons Usıng Cross-Lıngual Multımodal Pre-Traınıng
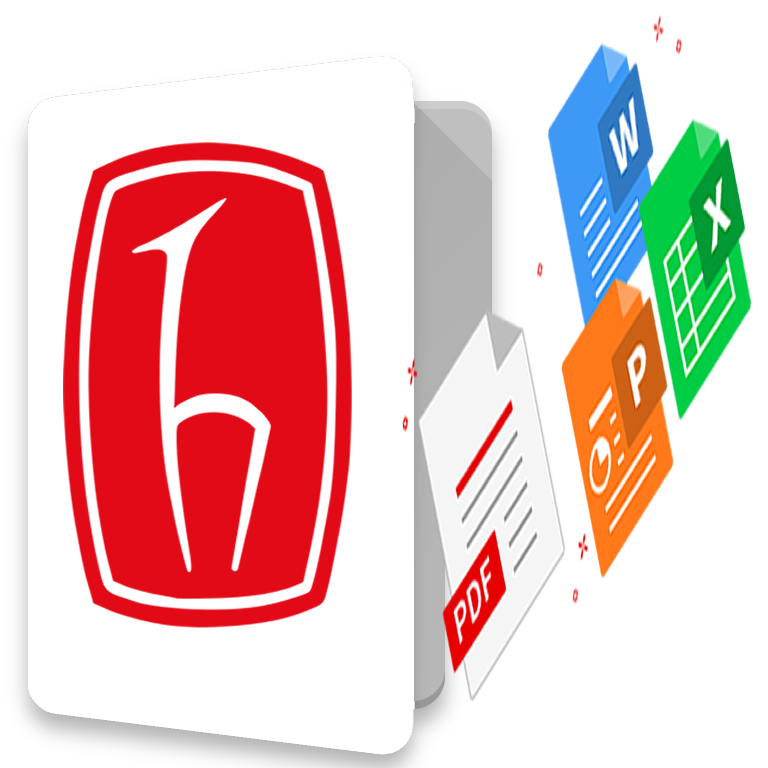
View/ Open
Date
2020-09Author
Kuyu, Menekşe
xmlui.dri2xhtml.METS-1.0.item-emb
Acik erisimxmlui.mirage2.itemSummaryView.MetaData
Show full item recordAbstract
In recent years, pre-training approaches in the field of NLP have emerged with the increase in the number of data and developments in computational power. Although these approaches initially included only pre-training a single language, cross-lingual and multimodal approaches were proposed which employs multiple languages and modalities. While cross-lingual pre-training focuses on representing multiple languages, Multimodal pre-training integrates Natural Language Processing and Computer Vision areas and fuse visual and textual information and represent it in the same embedding space. In this work, we combine cross-lingual and multimodal pre-training approaches to learn visually-grounded word embeddings. Our work is based on cross-lingual pre-training model XLM which has shown success on various downstream tasks such as machine translation and cross-lingual classification.
In this thesis, we proposed a new pre-training objective called Visual Translation Language Modeling (vTLM) which combines visual content and natural language to learn visually-grounded word embeddings. For this purpose, we extended the large-scale image captioning dataset Conceptual Captions to another language—German using state-of-the art translation system to create a cross-lingual multimodal dataset which is required in pretraining. We finetuned our pre-trained model on Machine Translation (MT) and Multimodal Machine Translation (MMT) tasks using Multi30k dataset. We obtained state-of-the-results on Multi30k test2016 set for both MT and MTT tasks. We also demonstrated attention weights of the model to analyze how it operates over the visual content.