An Enhanced Approach for Malware Detectıon By Utılızıng Computer Vısıon and Memory Forensıc
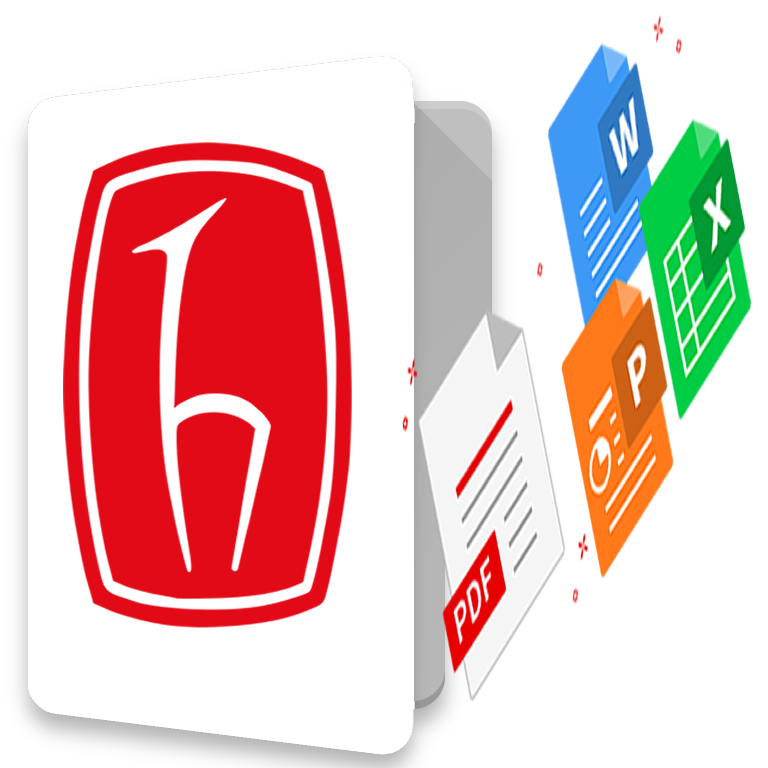
Göster/ Aç
Tarih
2020Yazar
Tahıllıoğlu, Erşan
Ambargo Süresi
Acik erisimÜst veri
Tüm öğe kaydını gösterÖzet
The number of advanced malware, which have been released, has increased substantially
year after year with the increase in the use of information systems. Due to the ransomware
programs, which we have frequently heard of in recent years, the effects of malware have
drawn attention. The detection and prevention of malware before resulting in destructive
effects have been a subject of research.
Malware detection methods are inadequate for detecting the sophistical malware to which
methods such as code obfuscation and packing have been applied. Portable executable
files (PE) leave some traces in the memory, and this allows for examining the behavior
of the malware by security experts.
In this thesis, malware were researched in detail, the studies conducted to detect malware
were examined and a new method was proposed to detect the sophisticated malware and
potential zero-day attacks. The proposed method in this study, dumped the memory patterns of the malicious process and then visualized them as RGB images in different
dimensions. The visualized memory dump data enabled the use of computer vision
methods. With the GIST and HOG global descriptors, which are frequently used in the
field of computer vision, feature extraction was performed over the created images and
vectors were obtained as a result of this process. These vectors were classified with
XGBoost, J48, Support Vector Machine, SMO, Random Forest machine learning
algorithms. In this study, the success of the visualization technique and different machine
learning algorithms in detecting malware was analyzed. According to the results of the
study, the highest accuracy was obtained when the visualization method with the fixed
width of 4096 was used. The success rate of this method was found to be 96.39%. This
study shows that the method proposed in the thesis can be effective in detecting malware.