Unsupervised Video Summarization With Independently Recurrent Neural Networks And Multiple Rewards
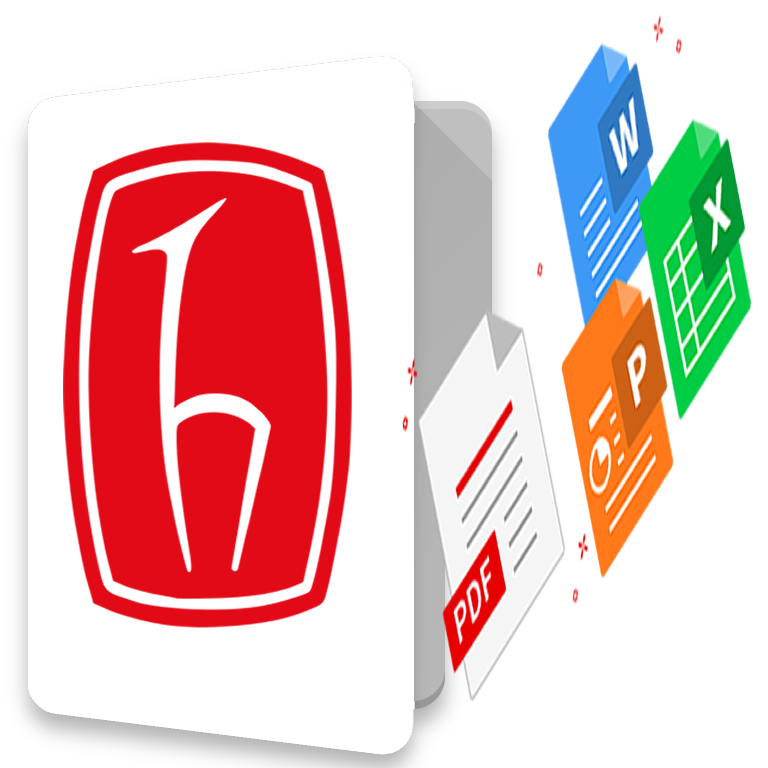
Göster/ Aç
Tarih
2019Yazar
Yalınız , Gökhan
Ambargo Süresi
Acik erisimÜst veri
Tüm öğe kaydını gösterÖzet
Video summarization, one of the interesting research areas that has significant acceleration in recent years, is producing shorter and concise videos that represent the content of long videos as diversely as possible. It is observed that sigmoid and hyperbolic activation functions used in long short-term memory (LSTM) and gated recurrent unit (GRU) models used in recent studies on video summarization task, may cause gradient decay over layers. Moreover, interpreting and developing network models are hard because of entanglement of neurons on recurrent neural network (RNN). Besides that, to create good video summary from long videos, a model needs to retain temporal coherence. Irrelevant jumps within key segments can confuse a viewer. Therefore, a model should compose video summary uniformly. To solve these issues, in this study, a method that uses deep reinforcement learning together with independently recurrent neural networks (IndRNN) is proposed for unsupervised video summarization. In this method, Leaky Rectified Linear Unit (Leaky ReLU) is used as an activation function to deal with decaying gradient and dying neuron problems. The model, which does not rely on any labels or user interaction, is designed with a reward function that jointly accounts for uniformity, diversity and representativeness of generated summaries. In this way, the model can create summaries as uniform as possible, has more layers and can be trained with more steps without having any problem related to gradients. Based on the experiments conducted on two benchmark datasets, it is observed that, compared to the state-of-the-art methods on video summarization task, better results are obtained.